Aritifical intelligence (AI) and machine learning (ML) is a trending topic in all technological domains. It offers a rich set of methods for data processing that can be used to solve practical problems, including those occurring in networks.
In this article we present a set of network issues for which the use of ML methods and different AI types can bring benefits and generally seems to make sense. Please note that this is a non-exhaustive list and is open to additions. For greater clarity, five main categories have been used. Also, the “Design-Build-Operate” model has been proposed as an additional perspective for classifying the network issues considered.
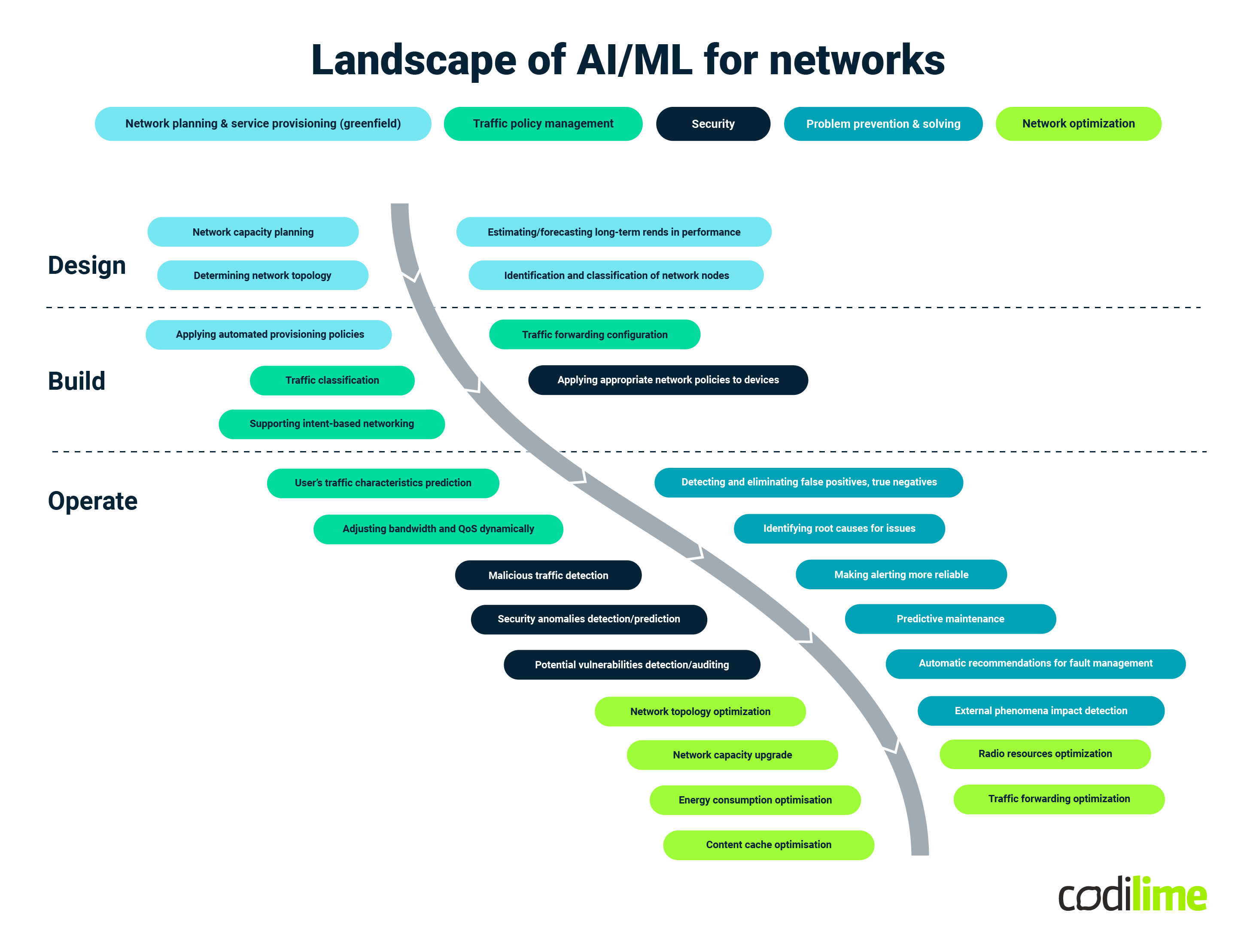
The network domains in which you can use AI & Machine Learning
These techniques offer many opportunities for data science and machine learning specialists to apply their expertise and solve issues in many aspects related to networking. Let’s examine the five specified categories:
Network Planning & Service Provisioning (greenfield)
- network capacity planning
- determining network topology
- estimating/forecasting long-term trends in performance
- identification and classification of network nodes (e.g. for IoT)
- applying automated provisioning policies (i.e. pre-configured traffic or security profiles for clients/devices)
Here, you can check our data science expertise in practice.
Traffic Policy Management
- traffic classification
- supporting intent-based networking
- user’s traffic characteristics prediction
- adjusting bandwidth and QoS dynamically
- traffic forwarding configuration (e.g. network load balancing)
Security
- applying appropriate network policies to devices
- malicious traffic detection
- potential vulnerabilities detection/auditing (e.g. due to outdated software, etc.)
- security anomalies detection/prediction (e.g. DDoS mitigation)
Problem Prevention & Solving
- detecting and eliminating false positives, true negatives (in alerting)
- making alerting more reliable (better than a threshold-based approach)
- identifying root causes for issues (multi-layer failure analysis)
- predictive maintenance (to prevent network degradations or outages)
- automatic recommendations for fault management (e.g. supporting network self-healing)
- external phenomena impact identification (mass events, ground works)
Network Optimization
- network topology optimization
- radio resources optimization (e.g. power-to-interference ratio optimization based on game theory)
- network capacity upgrade (e.g. predictive capacity tuning)
- traffic forwarding optimization (routes, paths, weights)
- energy consumption optimization (e.g. equipment switch on/off strategies, active/sleep scheduling)
- content cache optimization (e.g. content popularity assessment and proactive content loading)
Conclusion
We hope the above infographic will give you a better overview of where you can use ML and AI methods in the networks domain. Well-designed and implemented AI/ML techniques can benefit your network-based business. If you have questions about the practical aspects of AI/ML implementation for networks, feel free to contact us.