ABOUT THE AUTHOR
Katarzyna Hewelt
Data Scientist
Katarzyna is a Data Scientist at CodiLime with extensive machine learning and NLP skills and a strong passion for large language models and AI. She contributes to the tech community by sharing her knowledge through programming courses, articles on our blog, and by organizing TEDx Warsaw Women. Her background in various data roles gives her valuable insights into business needs. Katarzyna is skilled in data analytics, data engineering, and machine learning, making her an asset in her field. In her current role at CodiLime, she focuses on developing machine learning models, providing analytical support to clients, and creating innovative AI assistant solutions, including chatbots.
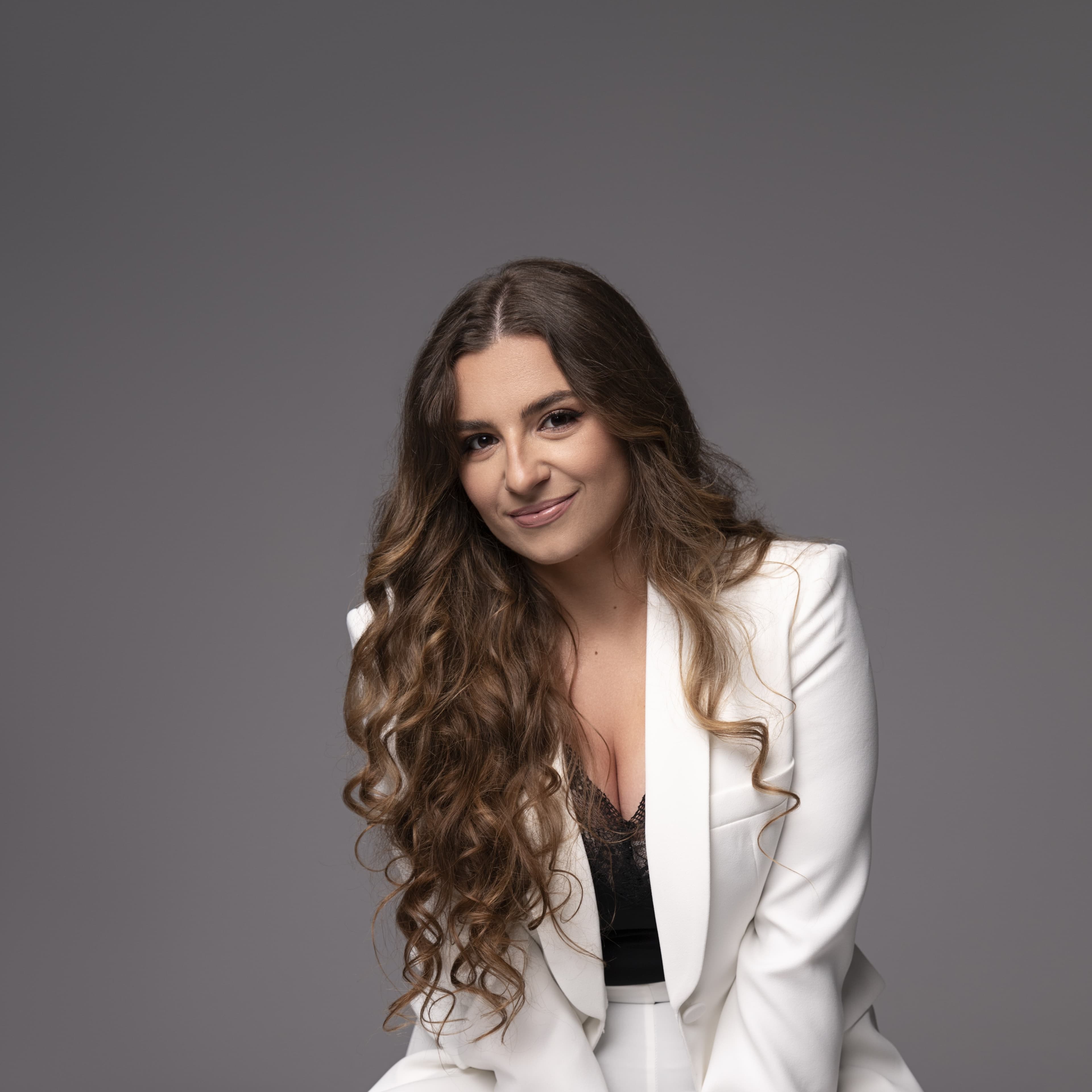
Recent posts by Katarzyna:
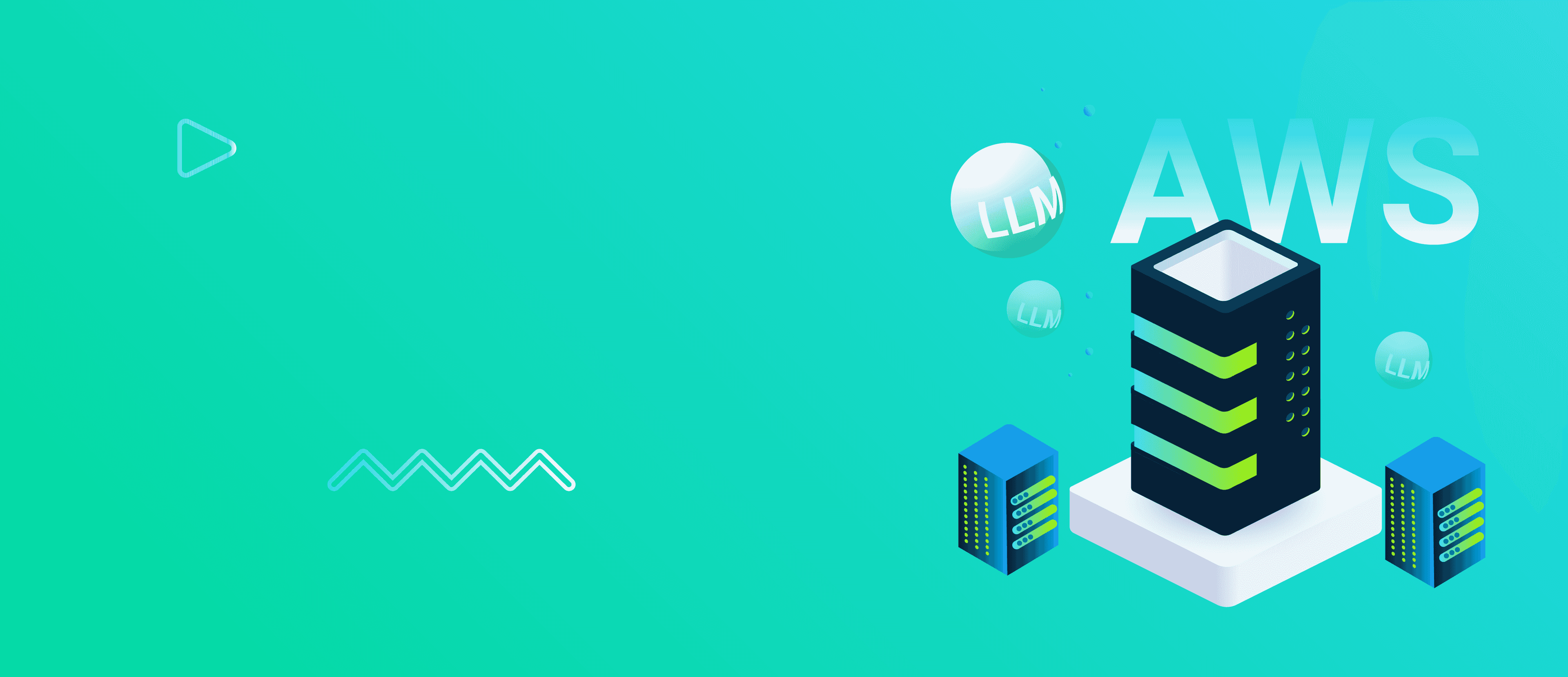
Hosting LLMs on AWS
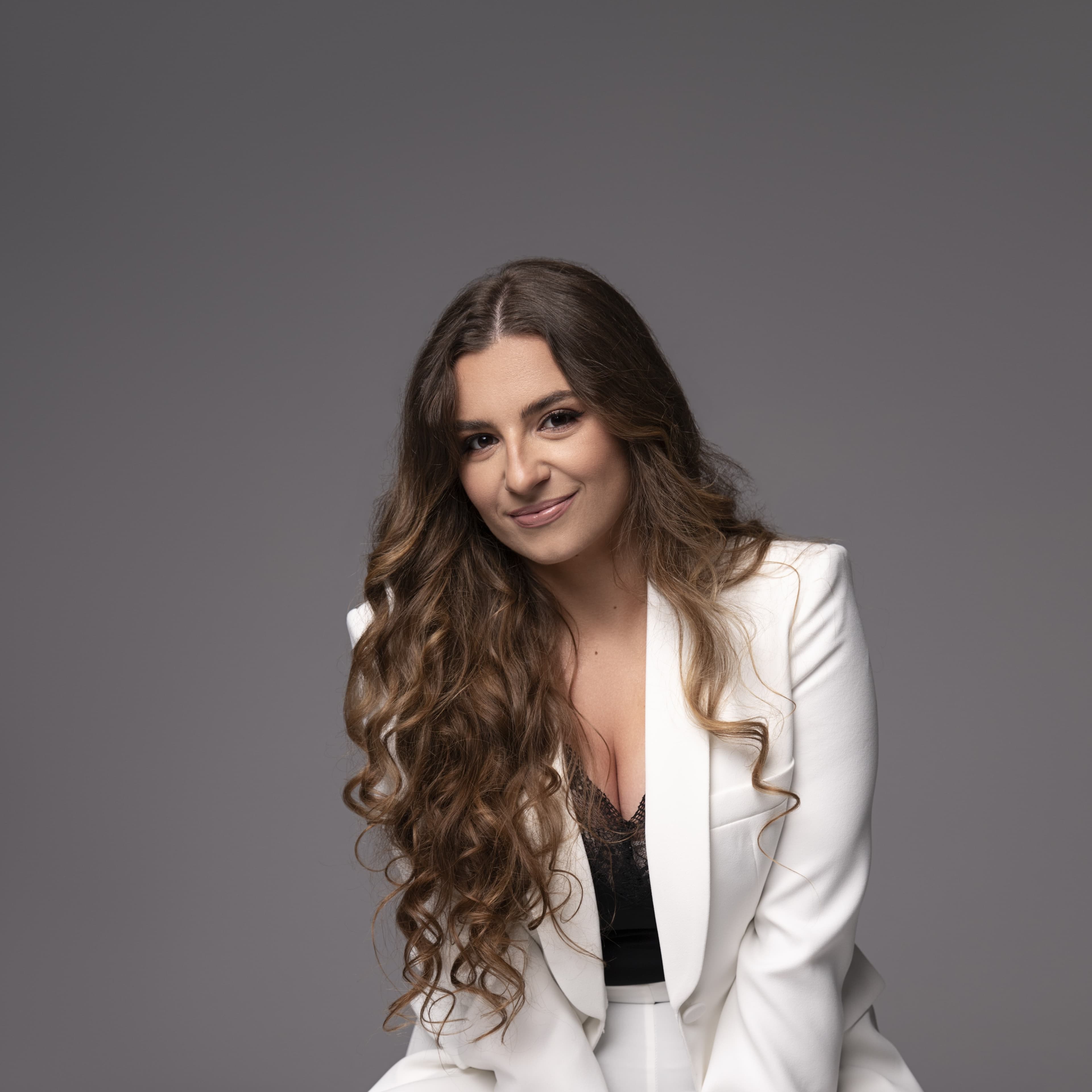
Katarzyna Hewelt
on Sep 24, 2024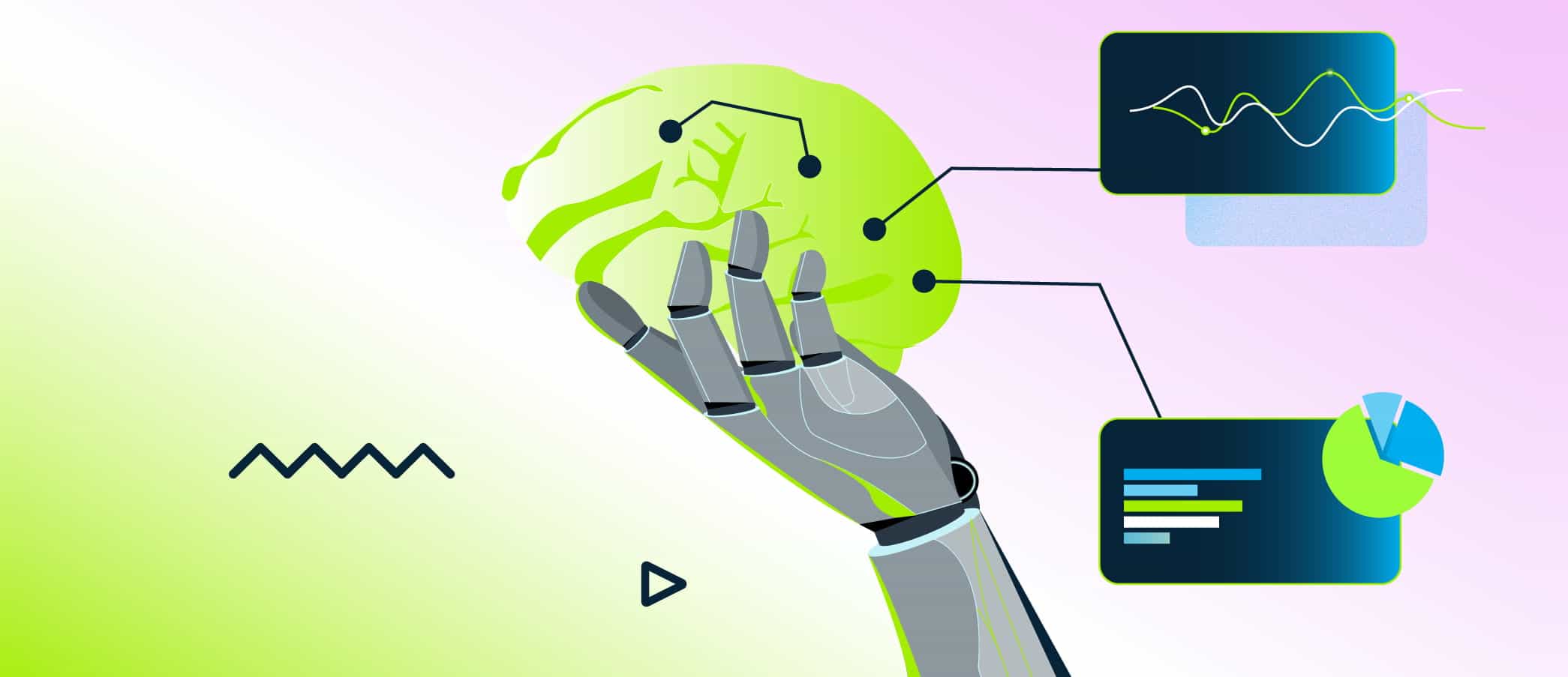
The crucial role of machine learning metadata and its influence on content embeddings
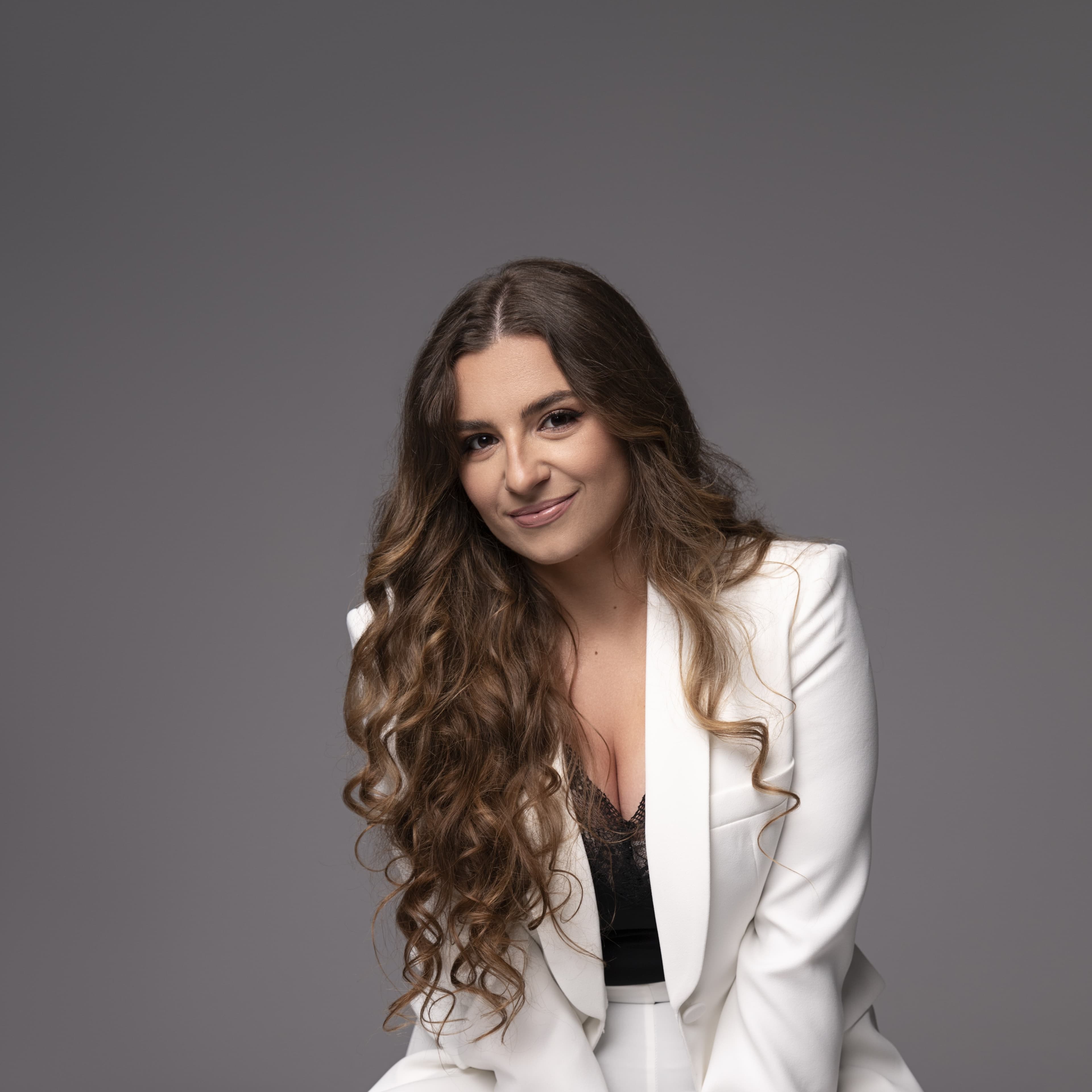
Katarzyna Hewelt
on Apr 9, 2024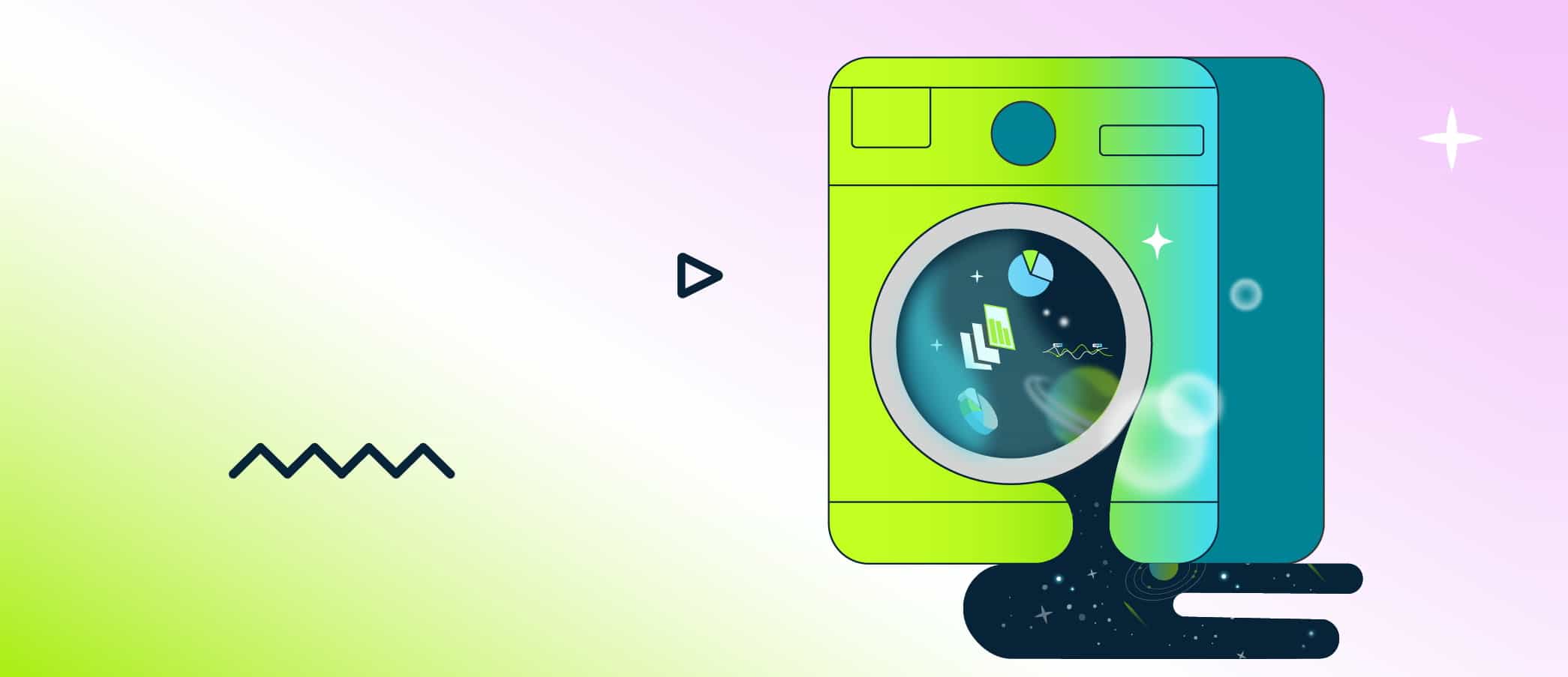
Data cleaning techniques: Strategies for reliable data analysis
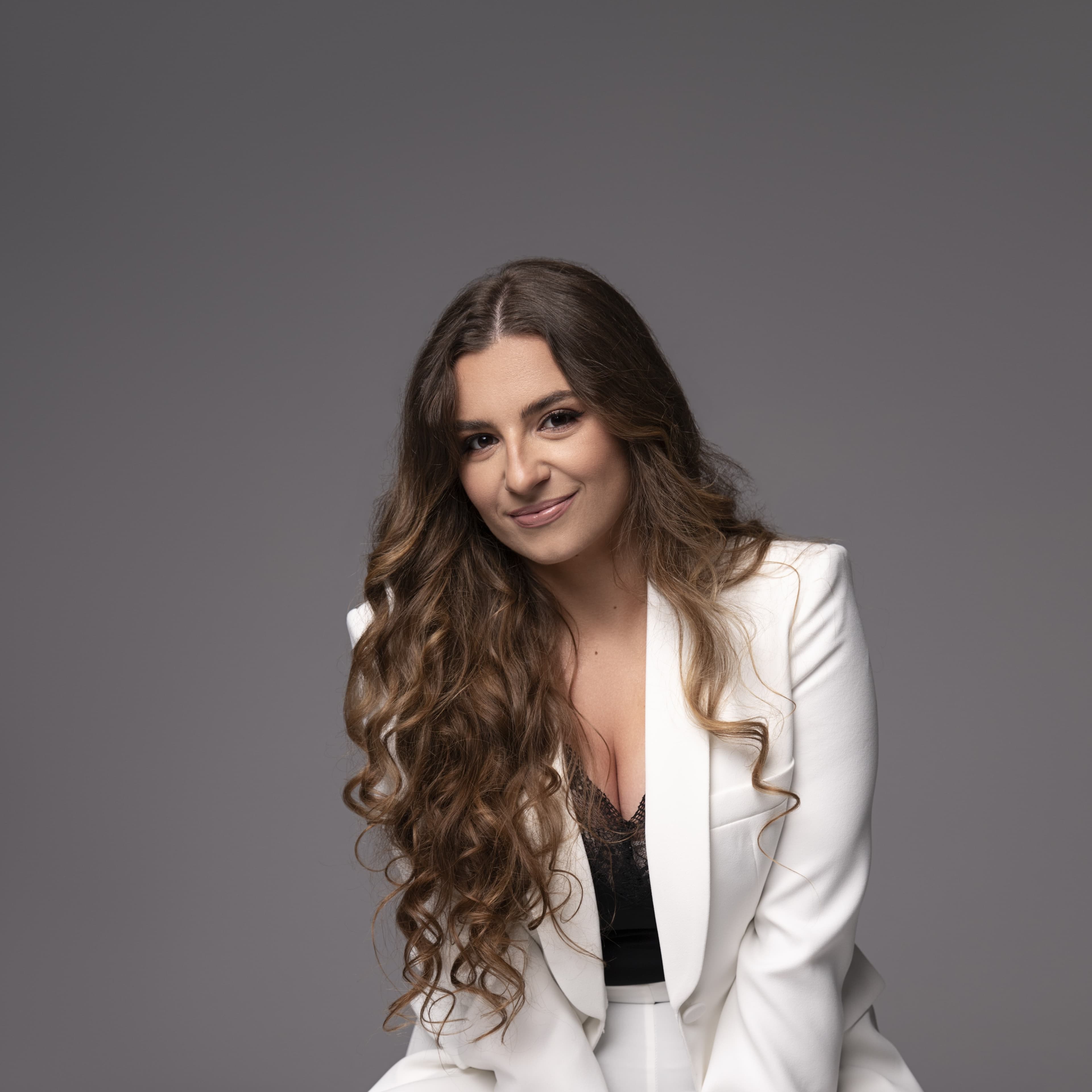
Katarzyna Hewelt
on Jan 3, 2024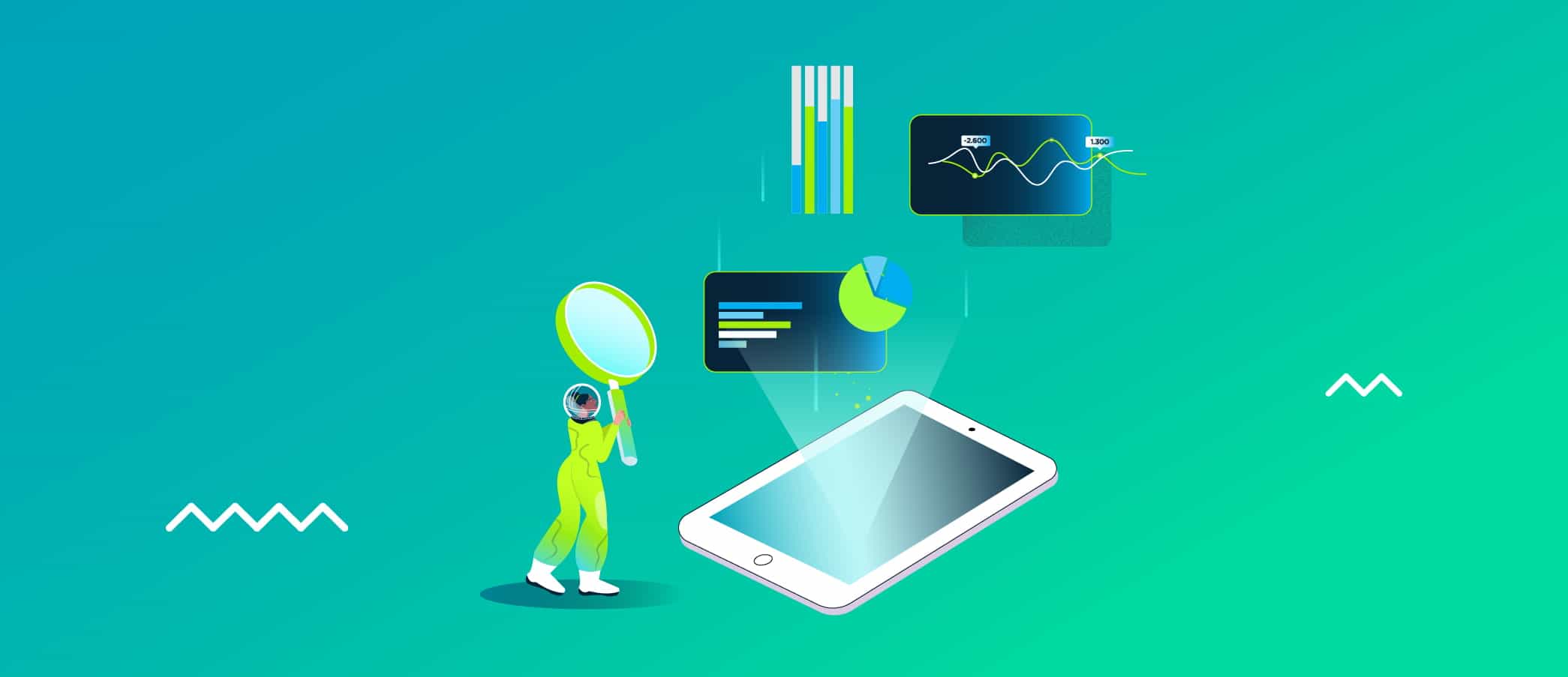
Understanding big data infrastructure: essentials and challenges
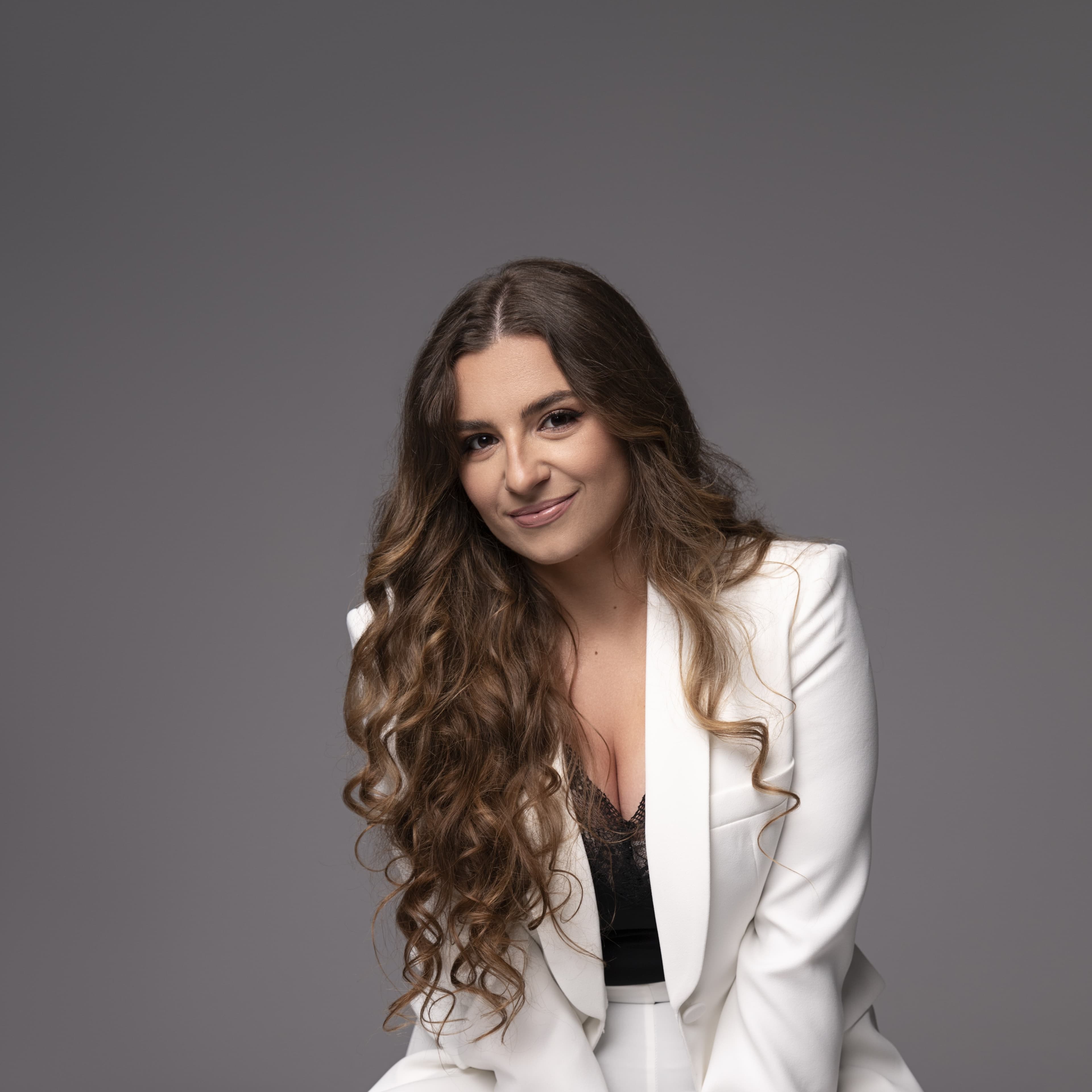
Katarzyna Hewelt
on Dec 7, 2023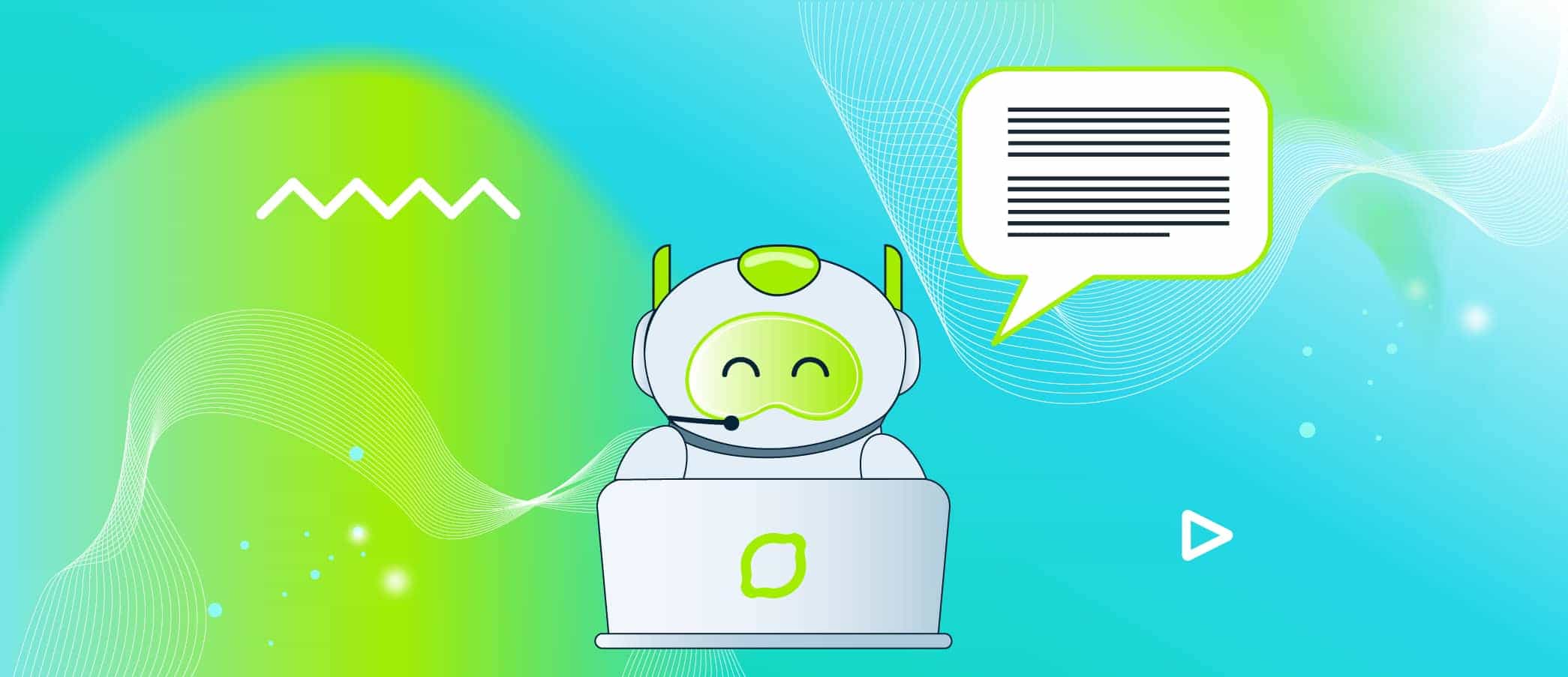
Private Slack Chatbot: an integration of corporate resources and large language models (LLMs)
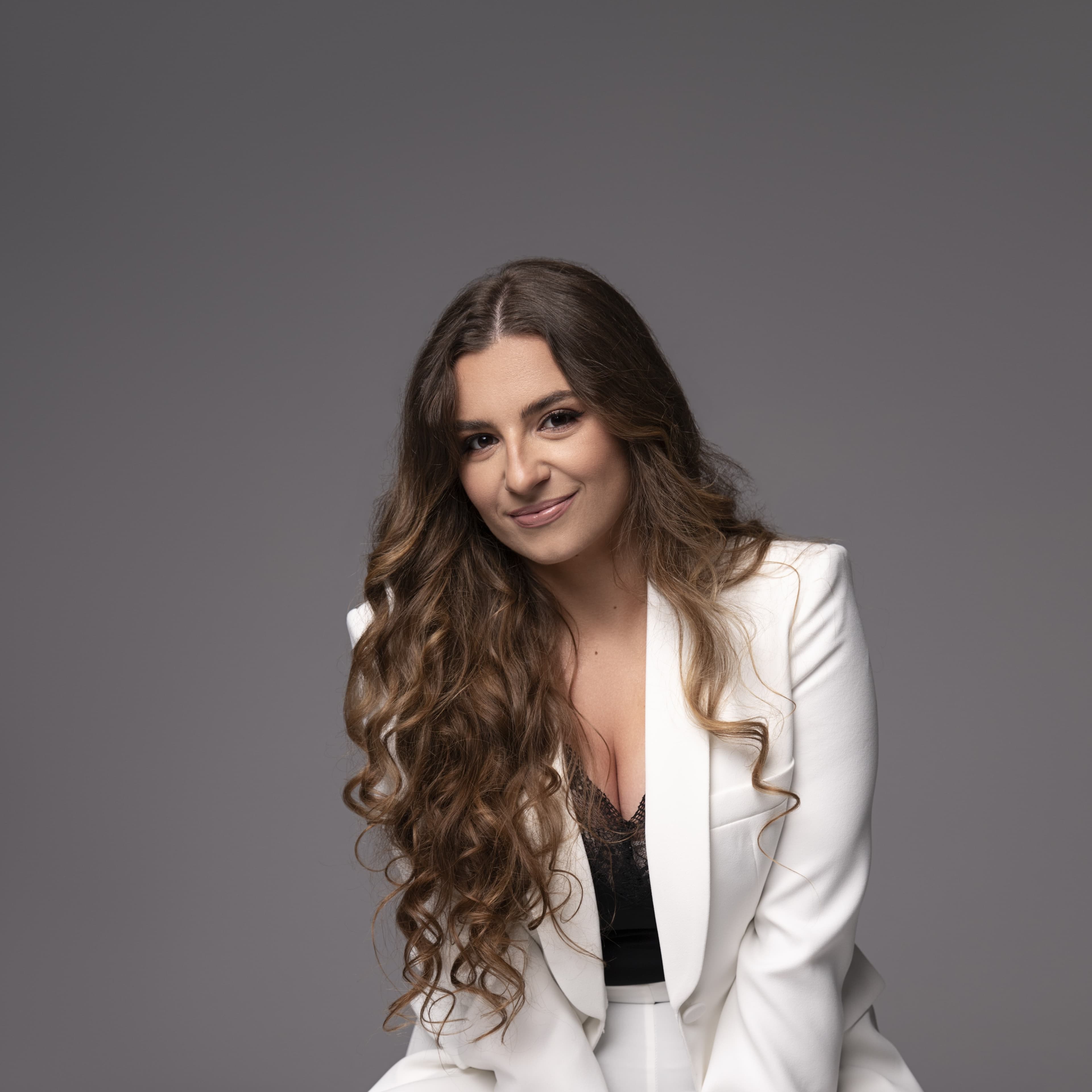
Katarzyna Hewelt
on Oct 19, 2023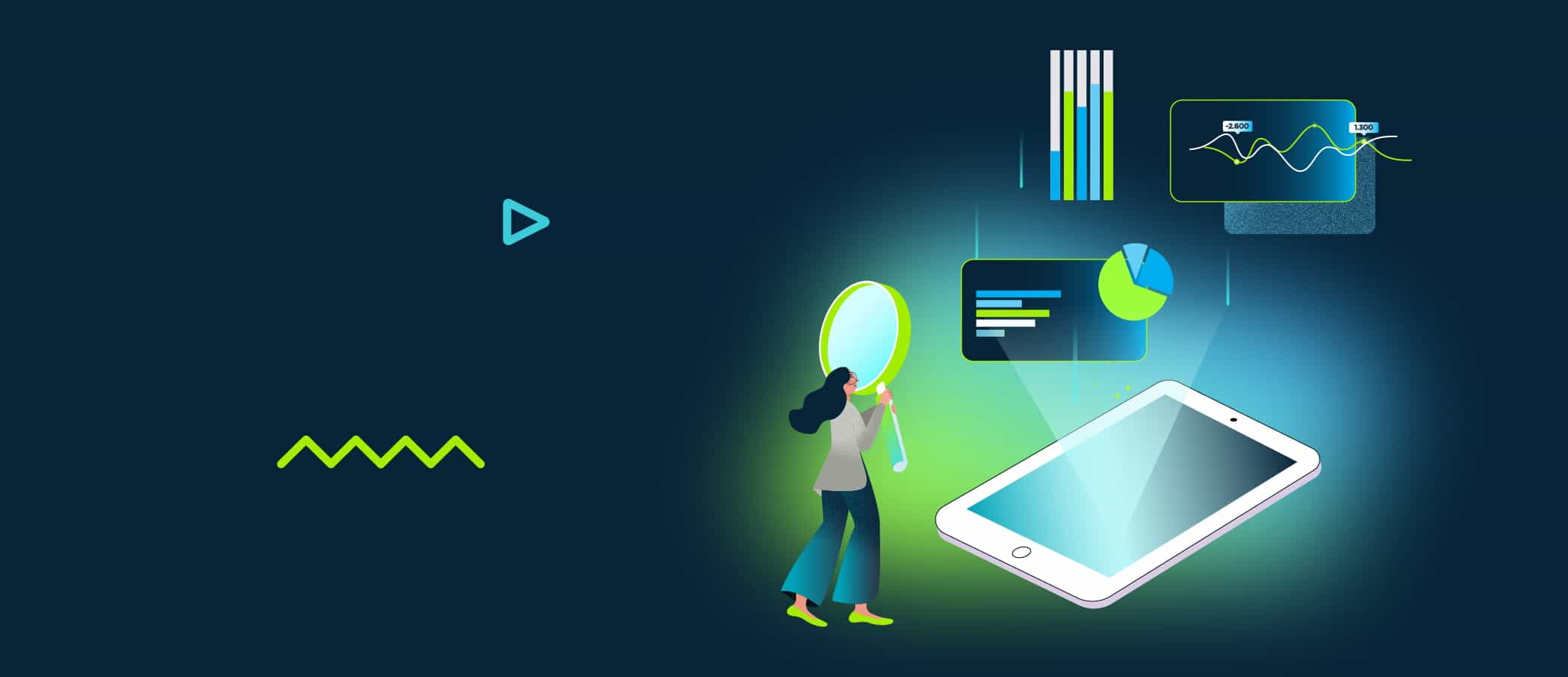
Detecting patterns, uncovering insights: the crucial role of data monitoring
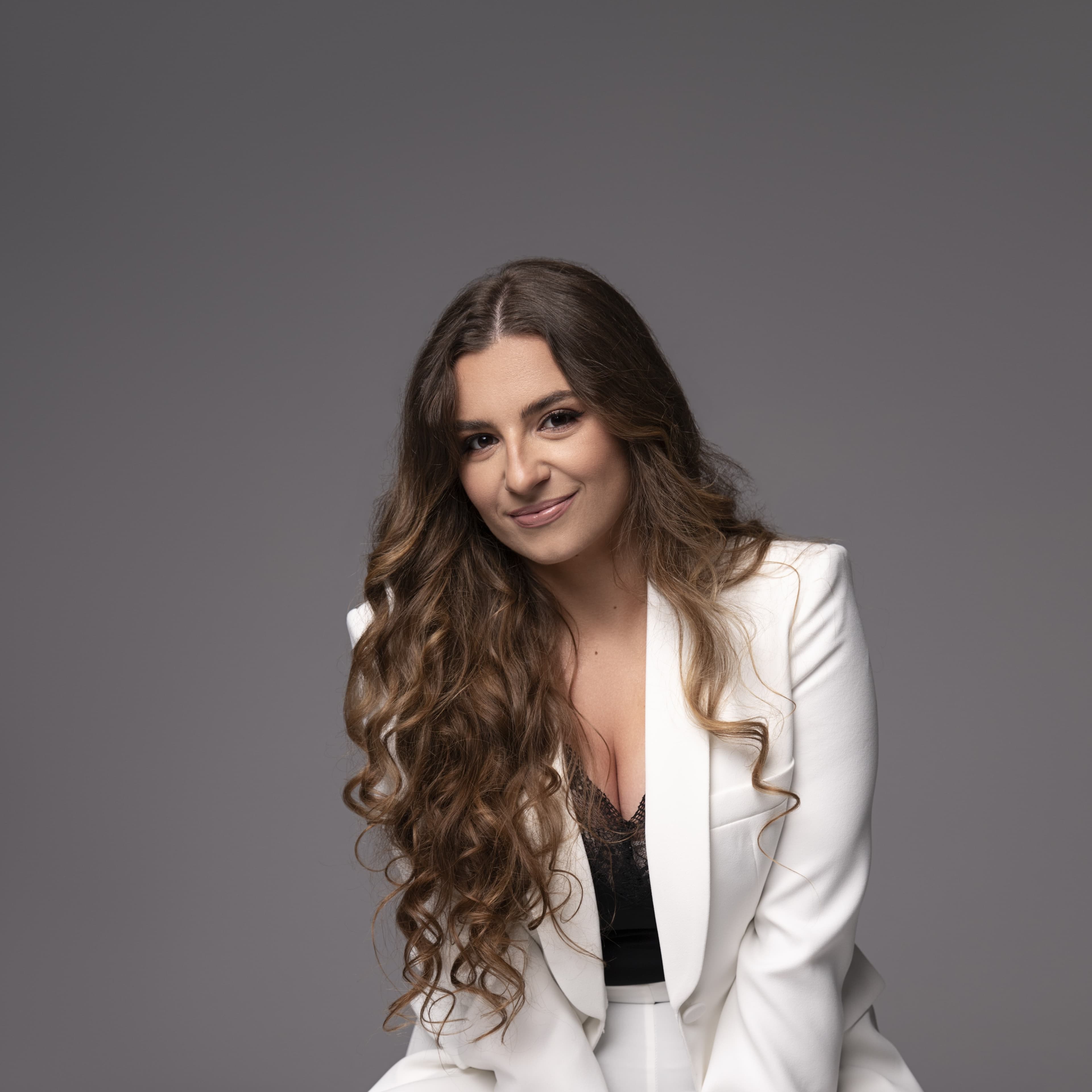
Katarzyna Hewelt
on Jul 7, 2023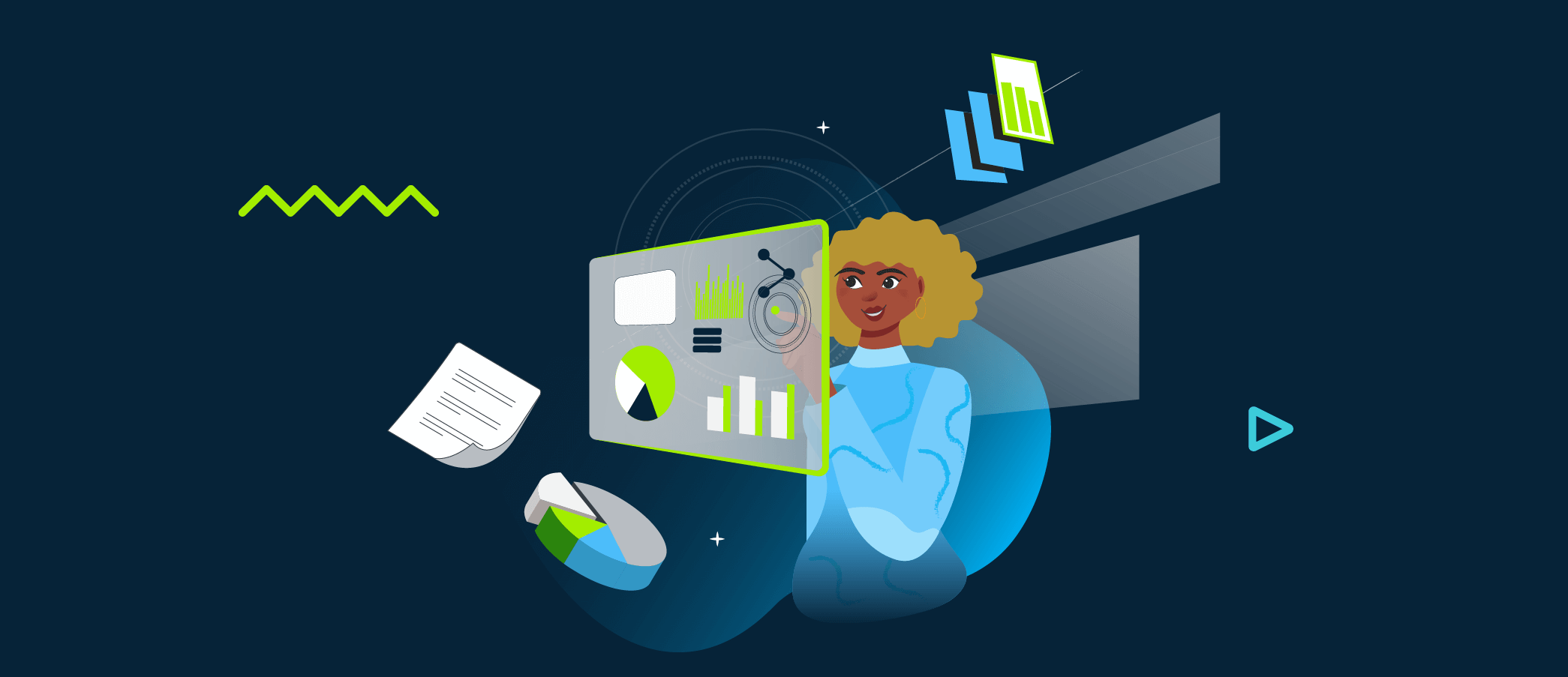
Data wrangling — what it is and why it is important
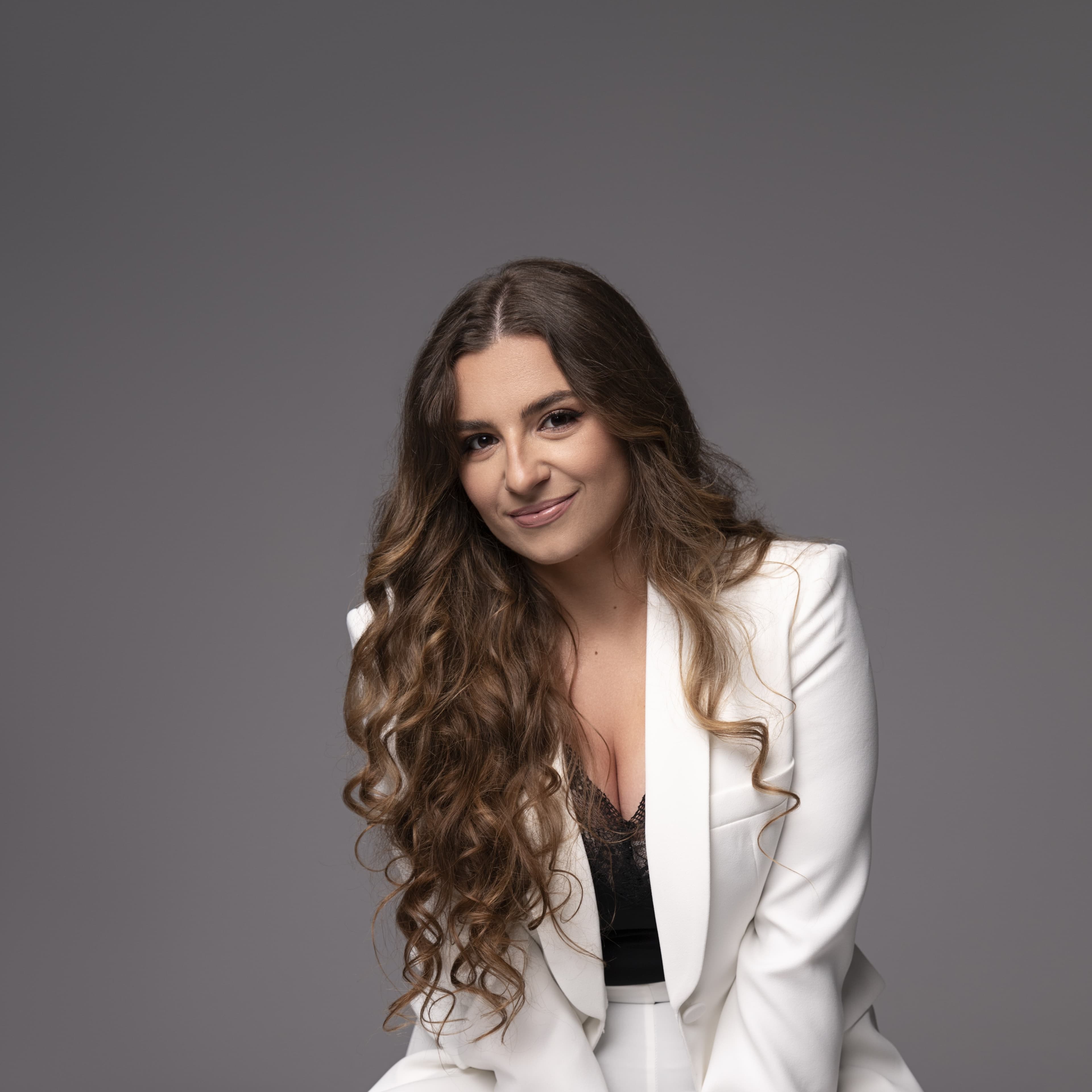
Katarzyna Hewelt
on May 8, 2023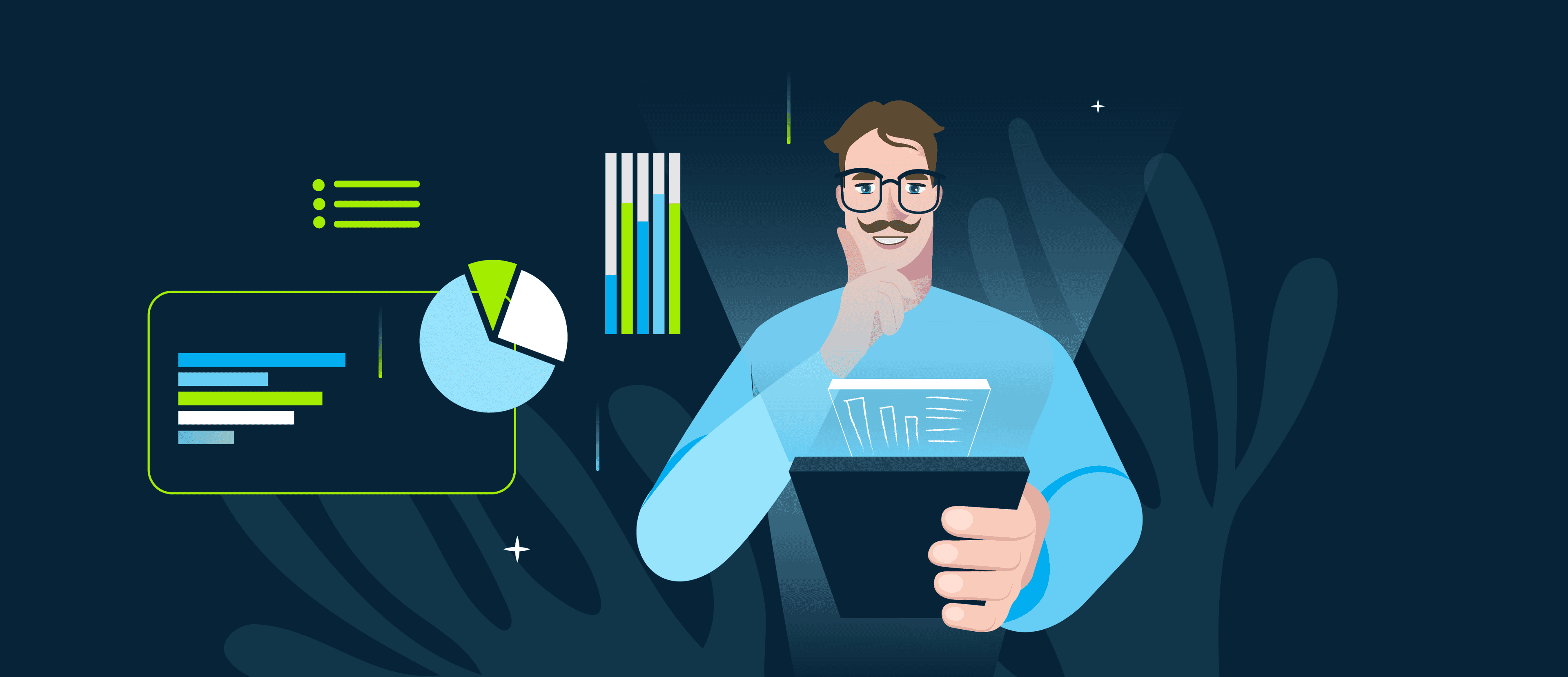
Data Science vs. Data Analytics — main differences overview
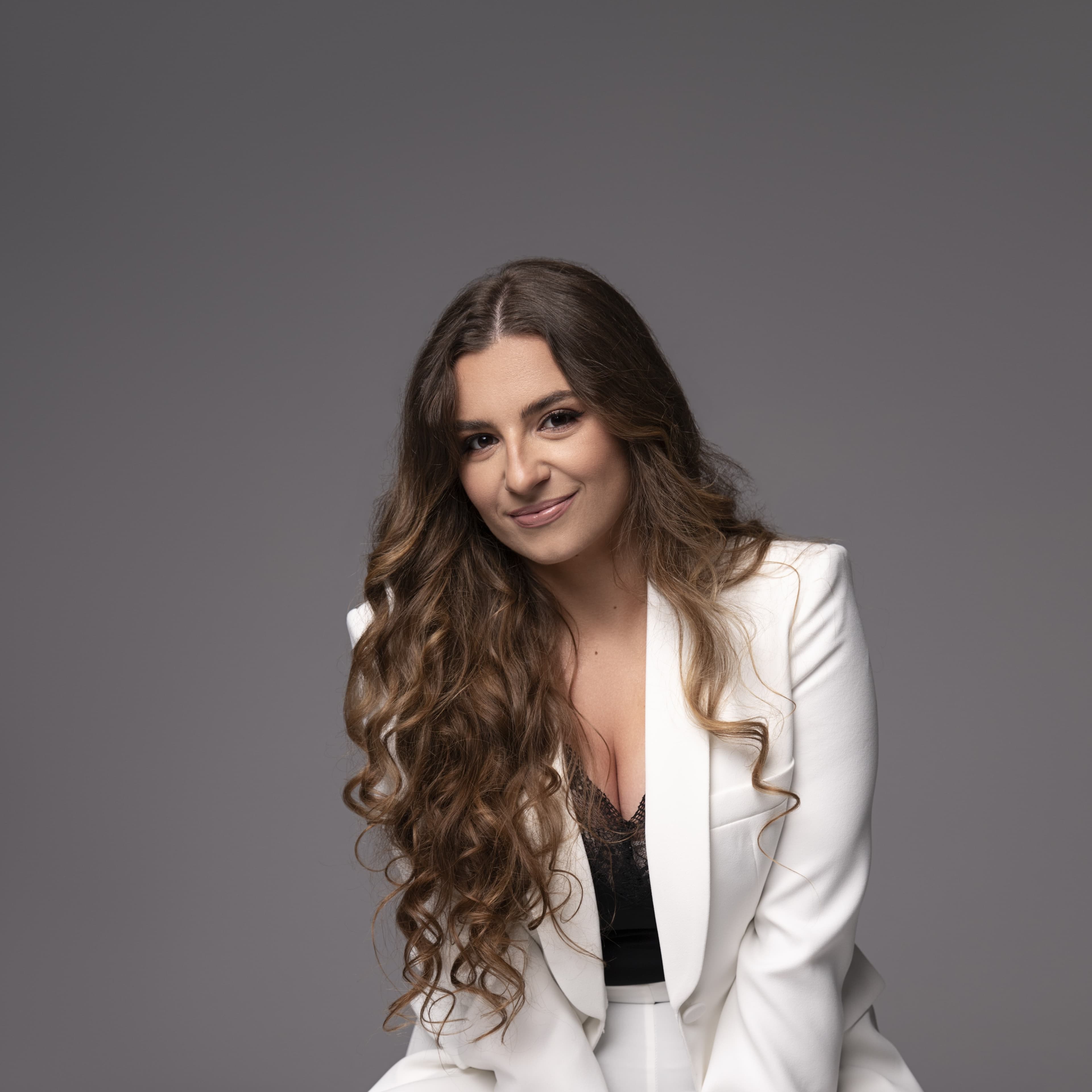
Katarzyna Hewelt
on Jan 26, 2023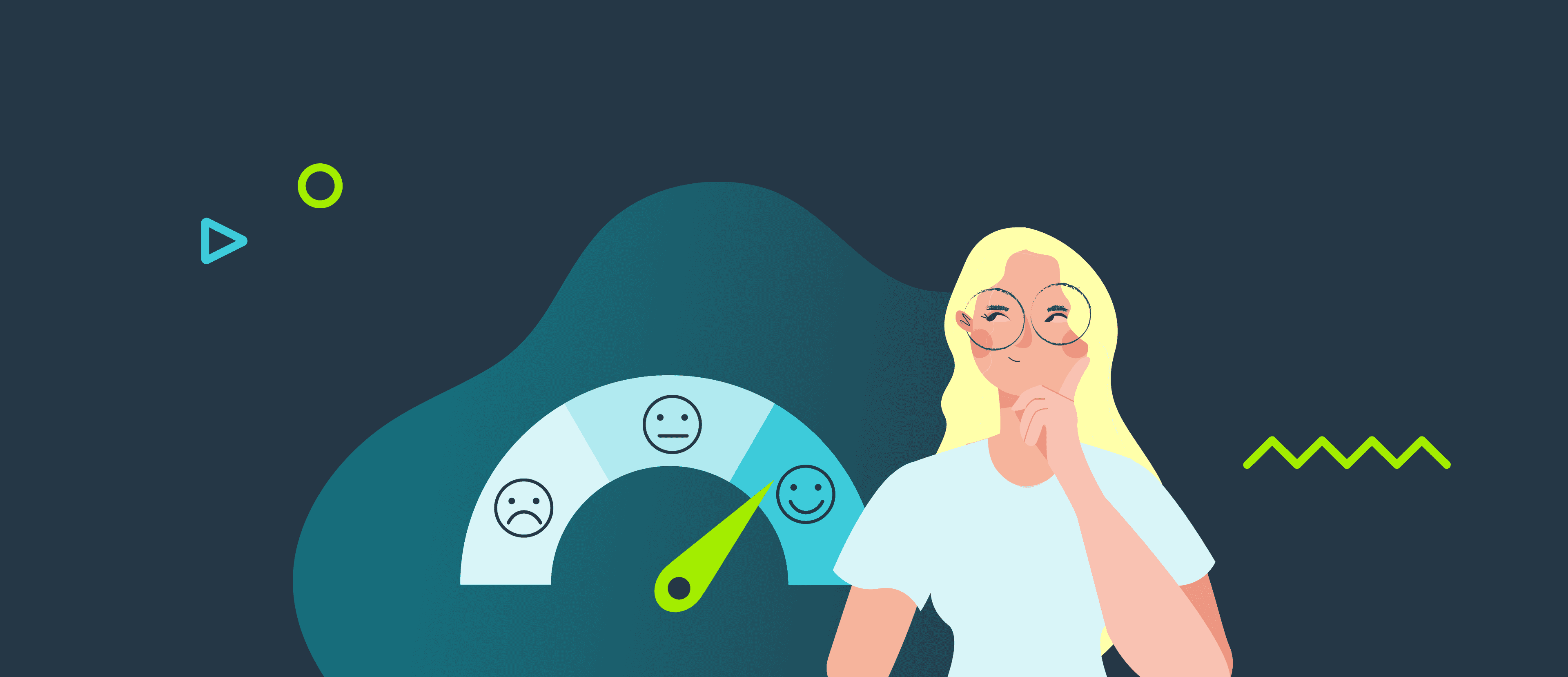
Sentiment Analysis. What is it and how to use it?
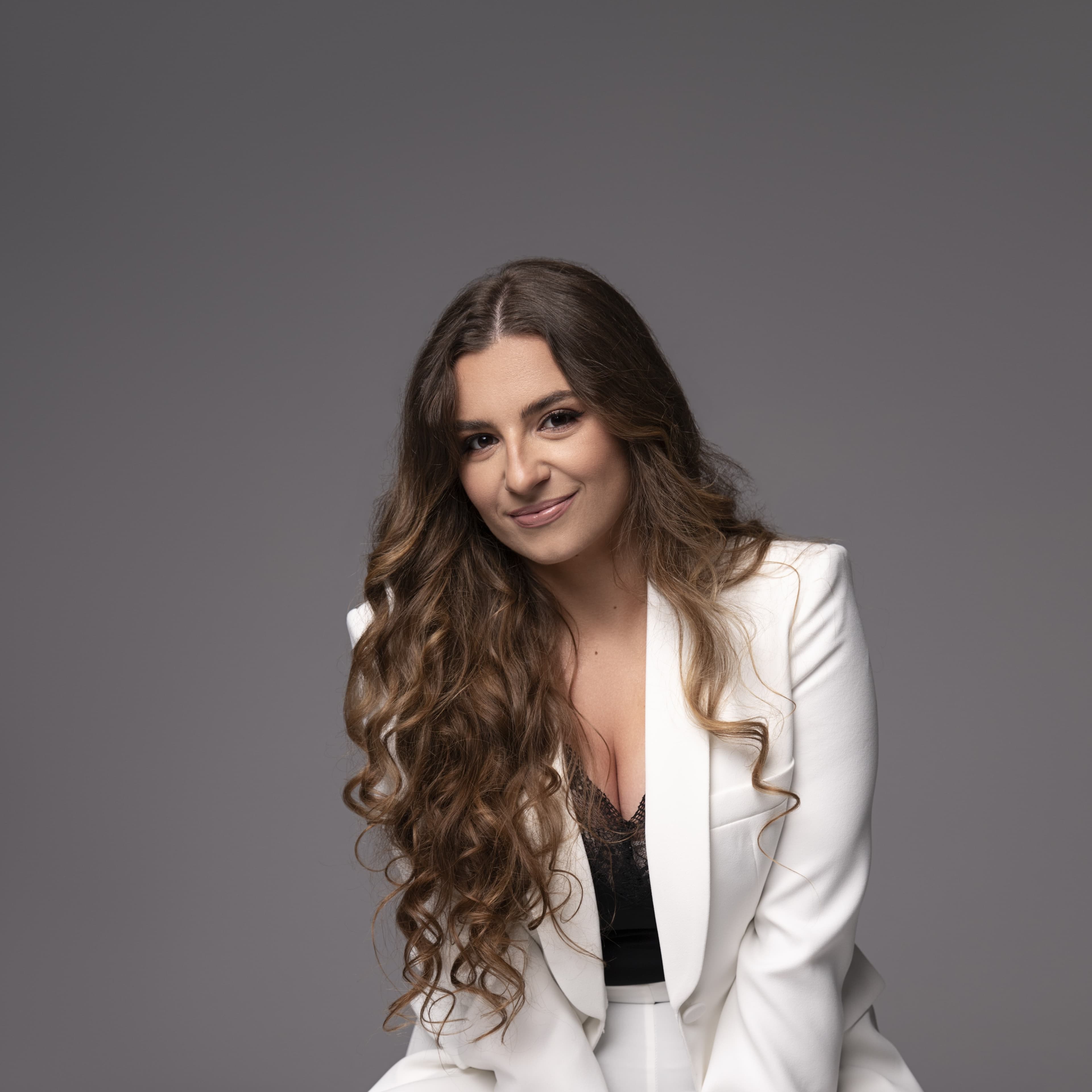
Katarzyna Hewelt
on Jan 10, 2023Get your project estimate
For businesses that need support in their software or network engineering projects, please fill in the form and we'll get back to you within one business day.
We guarantee 100% privacy.